Entwicklungsfeld III
Entwicklung und Erprobung von KI-unterstützten Lehr- und Lernangeboten
Durch die prototypische Entwicklung neuer Lernszenarien, -formate und -inhalte berücksichtigt das Projekt die Präferenzen und Bedürfnisse der Weiterbildungsinteressierten besser, steigert so die Bereitschaft und Akzeptanz für Weiterbildung und ermöglicht die Entwicklung individueller Weiterbildungsstrategien. Das Lernerlebnis wird realitätsnäher und trifft noch besser die Bedürfnisse der Zielgruppen.
Übersicht Ergebnisse
Lizenzierung & Nutzungsrechte
Zielsetzung der EduPLEx_API ist die Schaffung eines offenen de-facto Standards unter Open-Source Lizenz. Um Offenheit und Flexibilität in der Anwendung garantieren zu können wird auf ein international anerkanntes API-Format aufgebaut und dokumentiert.
Zugrundeliegende APIs werden offen und nicht kompiliert bereitgestellt. Der Source Code wird unter der MIT Lizenz (https://opensource.org/licenses/MIT) veröffentlicht. Damit wird die Wiederverwendung sowohl für Software, deren Quelltext frei einsehbar ist (Open Source), als auch für Software, deren Quelltext nicht frei einsehbar ist (Closed Source) erlaubt.
The language dimension of mental models
in digital learning environments
Mental models support learners to develop conceptual and operational representations of learning environments. They help learners to structure their knowledge and to translate learning goals for their knowledge acquisition into cognitive learning processes. It is essential for digital environments supporting self-paced learning not only to provide a clear picture of the knowledge the learner can acquire but also to help the learners in developing a clear image of their state of knowledge acquisition and to keep up a high level of learning motivation. This paper describes the human-computer interaction in the context of learning environments and the particular role of natural language in order to enrich this interaction.
How Natural Language and AI Raises Adaptivity and Individuality of Self-Paced Learning
Self-paced learning fosters the effective, efficient, and sustainable acquisition of knowledge and supports learning journeys adapted to the learners’ individual capacities and aspirations. There are many ways to engineer adaptivity for digital learning platforms. This paper presents design and prototypical implementation of a digital learning platform that uses AI-based analysis of natural language (NL) to engineer adaptivity for digital learning. NL provides many benefits for the communication layer of learning platforms. Learners appreciate the more natural interaction with their learning environment that, in turn, leads to a higher learning motivation and engagement. Natural language analysis (NLA) provides powerful methods and tools that help to summarize, categorize, and relate learning content. NLA produces annotations that foster the dynamic composition of learning components, like course chapters and related quizzes. Our NLA features include the application of nomenclatures like ESCO (The European Skills, Competences, Qualifications and Occupations taxonomy) as they provide unique and standardized vocabularies covering not only the learning components within and across learning materials but also descriptions of learners and their career aspirations. Our system enriches these annotations by adding further operable information from named entity recognition.
Prototypisches Lehrangebot zum Thema “Design Thinking”
Ein wesentlicher Mehrwert des Projektes entsteht durch die enge Verzahnung der datengetriebenen, plattformseitigen Innovationen insbesondere hinsichtlich Qualifikationsbedarfsanalyse und Lerntransferanalyse mit daraufhin angepassten Lerninhalten und Lernszenarien.
Auf diesem Weg sollen für die Branche stilbildende Lernszenarien prototypisch entwickelt werden, die nicht nur Wissen vermitteln, sondern den Kompetenzerwerb stärken.
Prototypisches kollaboratives Lehrangebot
Insbesondere steht hier die geeignete Verschlagwortung bzw. thematische Zuordnung der Eingaben und Eigenbeträge der Lernenden im Vordergrund. Dies ist insofern eine Herausforderung als das Feedback der Lernenden unterschiedliche Hintergründe haben kann.
Es können echte Beiträge sein, die für andere Lernenden hilfreich sein können, Hinweise auf (inhaltliche/technische) Fehler im Lehrmaterial, eine (positive/negative) Meinungsäußerung oder eine Frage zu den Lehrinhalten. Um das Nutzerfeedback korrekt zu behandeln muss eine zusätzliche Inhaltsbestimmung integriert werden, die das Nutzerfeedback zunächst korrekt klassifiziert.
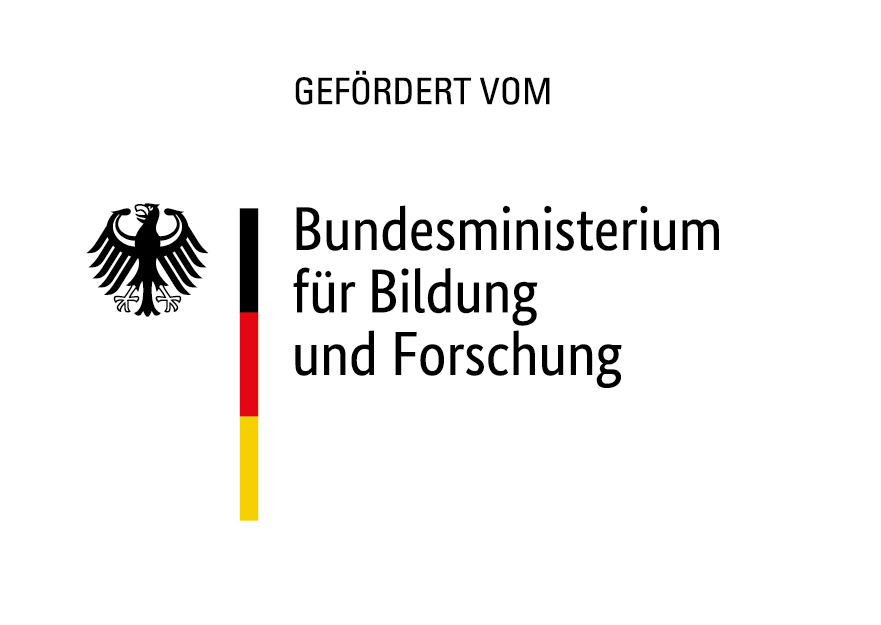
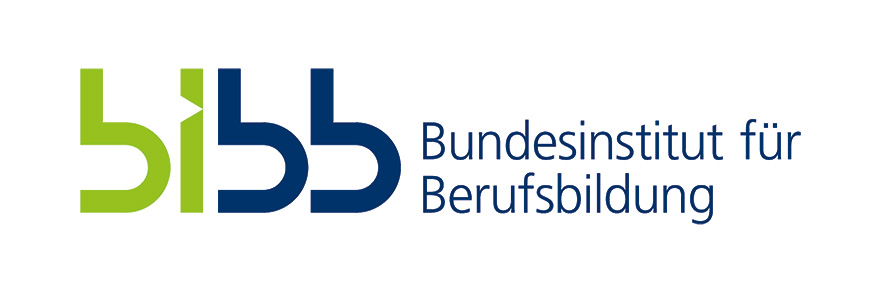
Kontakt
+49 (0)3683 688 4112
Prof. Dr. Regina Polster
Hochschule Schmalkalden
Fakultät Informatik
Blechhammer 4-9
98574 Schmalkalden
technische Fragen
DI Alexander Schmid
courseticket Deutschland GmbH
alexander.schmid@courseticket.com
+43 (0)660 778 779 1 (Werktags, 9:00 - 17:00 Uhr)